Inexpensive sensors with high-speed communication capabilities — described as Internet of Things (IoT) devices — have led to an explosion in data acquisition. However, data is of little value unless analyzed to yield useful actions. Many IoT sensors and devices send their data to the cloud for processing. Edge computing is an alternative to cloud-based processing that can benefit manufacturing in several ways.
What is edge computing?
Picture a network of sensors and computers, all sending data to and receiving instructions from a central server. In simple terms, this is how the Internet is organized. At the “edge” of this network are the IoT devices that interact with physical hardware. Pumps, motors, rolling mills, robots and packaging machines are just some examples of the machines that might be connected in an Industrial Internet of Things (IIoT). Every one of these machines could stream data back to a central server. This might include production statistics such as cycle time or operating conditions such as temperature and vibration. Inspection systems might be transmitting images for analysis and storage.
Such data can help operators monitor performance and identify improvement opportunities, but there are two limitations: latency and bandwidth. Latency is the delay that occurs when sending and receiving data. It can vary a lot, leaving cloud systems unable to perform real-time control. Bandwidth is the capacity of the “pipe” from device to server. As more IIoT devices are added to a system and sampling rates go up, the volume of data being transmitted grows. At some point the “pipe” becomes full and any additional data is delayed.
When discussing industrial IoT, edge computing refers to performing data processing at the machine or on the device. In some cases, this may require a computer on the machine, but more modern IIoT devices have edge computing capabilities. The volume of data transmitted is reduced because anything needing immediate response is handled directly on the machine.
How edge computing benefits manufacturing
Use cases for edge computing in manufacturing include:
- Gauging and inspection
- Production planning and control
- Process control and optimization
- Predictive maintenance
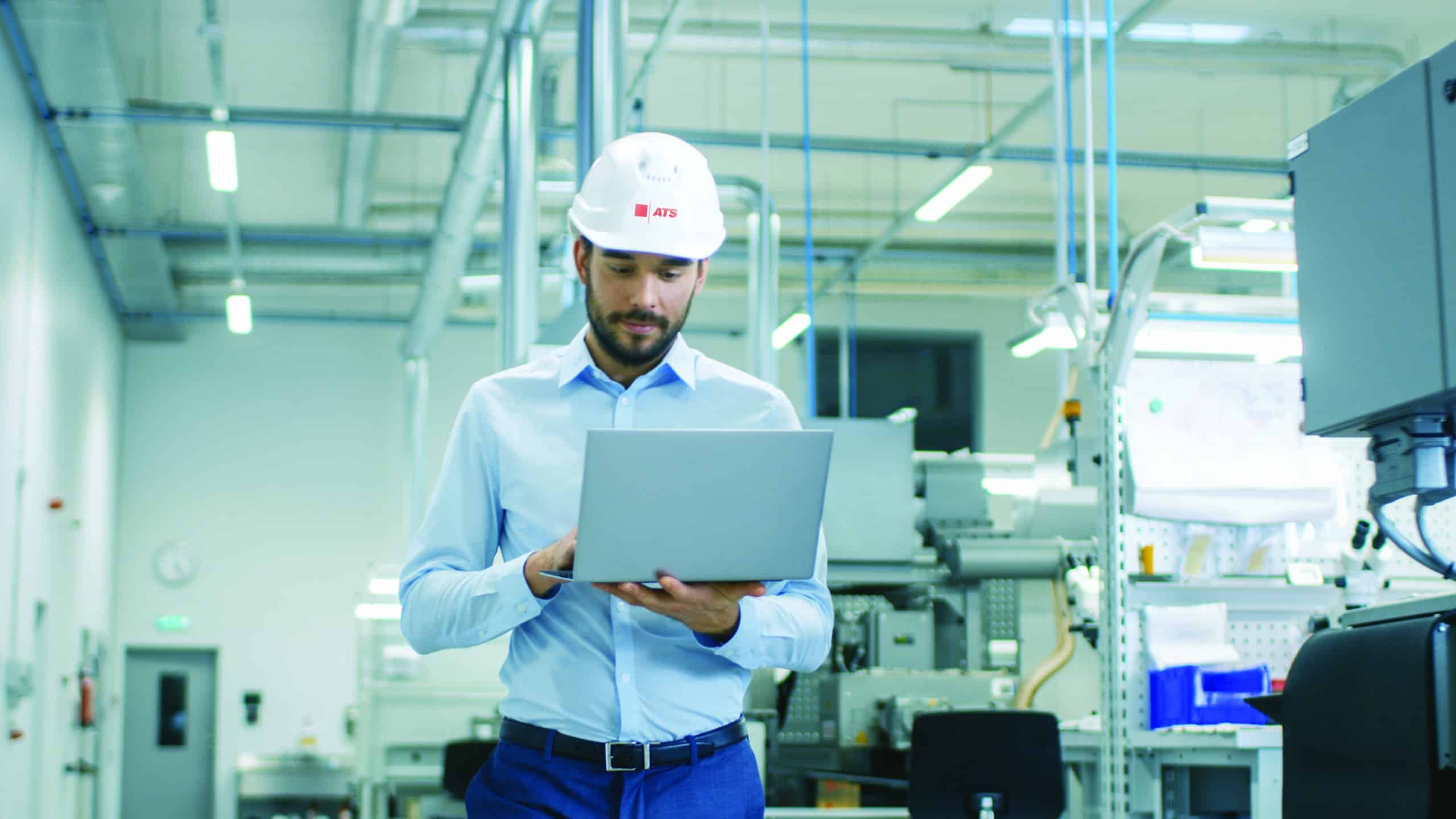
In gauging and inspection applications, industrial edge computing allows for analysis of data and immediate response to any issues observed. For example, a thickness measurement system might detect a drift and implement corrective action in a rolling mill or coating process. Image-based defect detection systems increasingly use artificial intelligence (AI) to find mistakes or excessive variability. This previously required the computing resources of a server but a growing number of edge computing IIoT devices can perform this at the machine.
Edge computing saves machines from reporting every aspect of production data to the server. Instead, the IIoT device might only report trends when limits are exceeded. Some manufacturing processes have large numbers of variables that make it a challenge to maintain product consistency. IIoT edge devices can monitor parameters such as viscosity, flow rates and temperature and use AI to optimize inputs in pursuit of an output goal. Industrial edge computing is especially useful for bringing analysis and response times as close to real time as they have ever been, thanks to less communication with a centralized server and in turn, a reduction in latency.
Smart factory edge computing addresses one of the most pressing needs of the connected facility, cybersecurity. With more localized processing, the facility can maintain more control and oversight over how data is handled. With local processing and onboard computers in newer equipment also comes a lower cost for centralized servers and related infrastructure, which can not only yield direct cost benefits but can also reduce energy and maintenance costs. The largest and most mature use of edge computing in manufacturing may be in predictive maintenance. This addresses one of the biggest challenges in manufacturing: how to eliminate unplanned downtime.
Edge computing and predictive maintenance
Many manufacturers combine reactive and preventive maintenance to maximize equipment availability while simultaneously attempting to minimize costs.
Reactive maintenance — running a machine until it fails and then making repairs — is a valid strategy in situations where downtime costs (lost output, quality problems, delayed deliveries, overtime working) are not excessive. However, when downtime drastically affects business performance, preventive maintenance is the norm.
Preventive maintenance involves taking a machine out of service on a scheduled basis and replacing components and adjusting in order to keep it running within specifications. The risk in this strategy is doing more maintenance than is needed to prevent breakdowns, and yet not doing the right maintenance.
Predictive maintenance offers an alternative. By using IIoT sensors with edge computing capabilities to monitor vital signs on manufacturing equipment — the mechanical equivalent of checking heart rate and blood pressure — it’s possible to detect and react to wear or faults before they affect production. Examples of the “vital signs” that can be monitored include:
- Temperature
- Vibration
- Current draw
- Fluid levels
- Flow rates
- Noise
- Speeds and cycle times
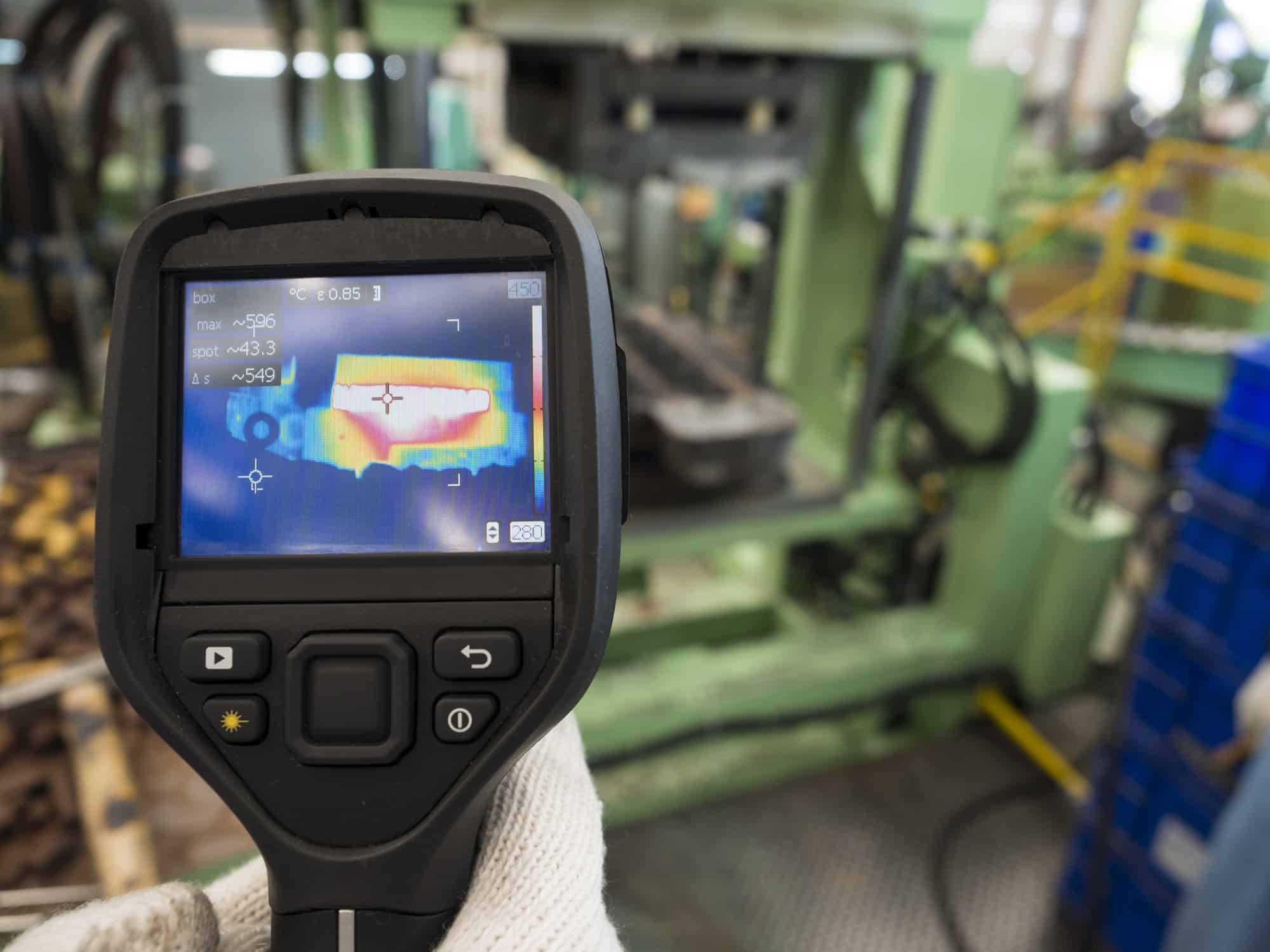
This type of monitoring can be applied in both discrete part and process manufacturing. Turning and milling centers, robots, and integrated assembly and packaging lines are all good candidates for predictive maintenance, as are mixing, reacting, processing, curing and coating processes. Wherever predictive maintenance is applied, the benefits can include:
- Improved equipment availability
- Protection against unplanned breakdowns and micro stoppages
- Reduced process and product variability
- Better safety
Industrial edge computing is a prime example of the potential of Industry 4.0 and the smart factory. With edge computing, Industry 4.0 foundations such as connectivity and responsiveness become all the more effective, with more bandwidth available for the most critical, data-intensive processes and more agility at the point of data collection to make independent, autonomous decisions. Edge computing analytics are one of the leading ways that the smart factory is becoming even smarter, approaching true real-time analysis and response.
Ask ATS about comprehensive maintenance solutions
As a leading provider of technology-based industrial maintenance, ATS understands the role that IIoT plays in effective predictive maintenance. The results are clearly measurable and include reduced unplanned downtime and higher productivity. Contact us today to find out how we could help improve the effectiveness of your maintenance operations.